This page provides BC faculty with CDIL’s guidance on how to approach the question of student use of Generative Artificial Intelligence (GenAI). It is not intended to serve as an official BC policy but rather as a clear outline of how CDIL recommends faculty answer this question.
The Challenge
In an AI world, academic integrity presents a particular difficulty for faculty. Specifically, how can they maintain academic integrity in their teaching while maintaining a positive relationship with students based on trust and scholarly work to help them achieve their learning goals?
The Solution
CDIL recommends a two-fold approach:
- Purposeful assignment and assessment design; and
- Clear communication with students on the role of AI in your course and a clear explanation for why you have taken this position.
This is not to say that it provides faculty with a ‘silver bullet’. Rather, it provides guidance that is part of a wider conversation that CDIL is facilitating on AI and Teaching.
But What About Plagiarism Software?
Unfortunately, it is not a viable solution. There are three reasons why:
Accuracy
Given the nature of Generative AI as a technology and its basis in large language learning models, it is impossible to prove whether or not work has been generated by AI. Plagiarism software companies themselves acknowledge the high rate of false positives in their detection of AI use in student work.
Equity
Several widely used tools, such as Grammarly or text-to-voice, have used AI technology for years. These tools are generally acceptable, increase educational accessibility and are crucial for many marginalized student groups’ participation in education.
FERPA and Intellectual Property
Running student work through AI detection software potentially puts their content into the public domain and could inadvertently create concerns regarding FERPA and intellectual property. As a result, Boston College generally does not endorse the use of plagiarism detection software due to its potential risk to student intellectual property and creating an adversarial relationship between faculty and students as it relates to academic integrity. BC service units will be unable to provide technical assistance or guidance to faculty who independently opt to use plagiarism detection software.
So how do faculty uphold BC’s Academic Integrity Policy? The policy has been updated (May 2024) to include specific language around the use of AI. The information below provides guidance on how can faculty be sure that students follow along with these policies.
Where Do You Start?
This guide is intended to equip faculty with approaches and guidance that are more equitable and more effective in maintaining academic integrity. While AI presents a new technological challenge to faculty, there are plentiful, proven well-established approaches to deal with it.
1. Explore AI for yourself.
At the time of this writing, Summer 2024, there is a high degree of variation in the ‘quality’ of output from Generative AI for each academic discipline.
In some disciplines, such as Computer Science, the output is high quality up to a certain point. For others, such as History, where the core of the discipline requires questioning popular generalizations, the output can be factually incorrect and amplify problematic historical accounts.
Your first goal should be to understand the basics of how it works and how it works in relation to your academic discipline.
2. Talk to your students
The most important thing you can do when approaching GenAI in your teaching is to establish and maintain an ongoing dialogue with your students about GenAI. The best course of action involves a multipronged approach:
Communicate your expectations in a written statement in your course syllabus and in person as a facilitated in-class discussion. Educational Technology writer Lance Eaton maintains a large repository of AI syllabus statements across a range of disciplines.
Facilitate an in-class discussion about GenAI with your students. This is extremely important. Focus on your experience with GenAI and how, as a disciplinary expert, you see it impacting student work and learning for your course.
Professor Sylvia Sellers-Garcia of the History department has an excellent blog post on her experience of facilitating this discussion. And our other colleagues at CDIL have posted about their own experiences as well.
You should aim to have a dialogue– where you share your perspective as a disciplinary expert and students share their perspectives on GenAI. In particular, connect your findings to your course’s learning goals and expectations.
We have encountered many faculty members who believed their syllabus statements clearly banned the use of AI tools in student work. But without an open dialogue, students’ conceptions about what constitutes an AI tool did not match those of faculty members.
CDIL has developed a system of visual communication for clearly explaining expectations around student use of AI for different assignments. Professor Rita Owens of CSOM has used these in her courses.
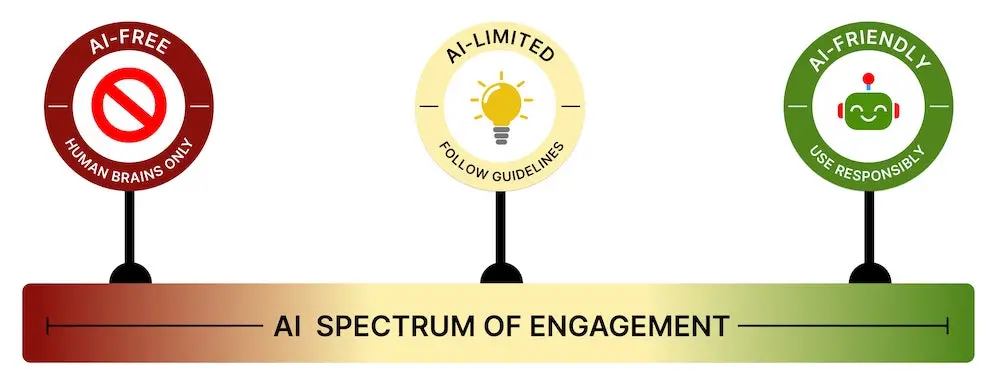
3. Review your course learning outcomes.
Considering how the availability of AI tools impacts your course learning outcomes is challenging and requires considerable thought, and it will, in turn, impact what kinds of assessments you task your students with. In the following section, we provide guidance on how to approach it.
Ways to Revise Outcomes and Assignments
What kinds of learning outcomes and assessment design facilitate academic integrity in an age of AI?
Communicate Clear Learning Goals
When thinking about your assessments in the age of AI, you need to start with your learning outcomes and develop precise goals for student learning.
Having specific goals helps you clarify your communication with students about the role of AI in the course. Some learning outcomes might be AI-agnostic, so you might allow students to use AI freely. Other learning outcomes might be achievable instantly by using GenAI. Here you have a decision to make: Do you change the learning outcomes and devise new ones focused on what AI can’t do? Or do you keep the learning outcomes as they are because students need to be able to do them as they are fundamental to their success in the discipline, regardless of whether AI can do it or not?
Design outcomes that require students to meet benchmarks that AI cannot accomplish. This could look like personal reflection, deep critical reflection, evaluating content, consistent writing voice within a single assignment, dealing with original or highly contemporary scenarios, working in teams, building social-emotional connections, or navigating scenarios with great ambiguity and complexity.
This approach removes you from evaluating whether students used AI in their work. Rather your focus is on evaluating if they met the expectations for the work or not, and being evaluated on that alone.
See below for a selection of rubrics and different approaches to grading from BC faculty that have explicitly been redesigned by faculty with AI in mind.
- Professor John FitzGibbon, MCAS Undergraduate: Populism and the Rise of Anti-Democracy
- Professor Cassidy Allen MCAS Undergraduate: First Year Writing
- Professor Drew Hession-Kunz CSOM MBA: Finance
Clarity and certainty about what you want students to learn in your course empowers you to articulate what types of assessments you require them to demonstrate their learning. The availability of GenAI technology means that faculty need to rethink their assessments away from traditional formats.
Emphasize processed-based, authentic Learning
In particular, process-based and project-based approaches to learning emphasize students’ ability to acquire and demonstrate mastery of skills and knowledge through active engagement with a complex problem or project.
In these approaches, the final “product” is less important than students’ ability to articulate their metacognitive awareness of their process and work.
Students learn the course content through the process of creating something, which requires them to apply the skills and content of the course material.
Because students have to create something new, they must demonstrate complexity in their mastery of skills and knowledge of any course at the highest level of Bloom’s taxonomy– “create.”
While GenAI is adept at producing text, images, and video within seconds, it cannot demonstrate the long-term reflection and articulation of cognitive development required in process—and project-based approaches to learning.
Focusing on a course’s final product necessitates learning outcomes structured around the metrics of “failure” or “success.”
Creating regular activities that scaffold learning for students by building toward the final product or giving them repeated practice at the skills needed in the process/project-based assessment helps students not only to build mastery but also underscores relevance for students. For example, consider a common assessment that students often think is “busy work”– the discussion board post. If students don’t see the connection between this learning activity and larger course outcomes or skills, there’s a huge temptation to offload this work to GenAI. Discussion board posts can be powerful to prepare students for class discussion, check their comprehension of course material, or provide an opportunity for reflection and connection of class materials to their lives outside the classroom. However, if students feel that this work is for the professor, it’s easy for them to see it as being performative in nature. However, if students clearly understand why they are being asked to complete this work and, more crucially, how this work will help them be successful, they are less likely to feel tempted to turn to GenAI.
How do product vs. project or process-based approaches differ?
Teaching from a process-based approach doesn’t mean eliminating “traditional” assessments like the research paper. However, the framework for assigning this assessment differs.
Product-Based | Process or Project-Based | |
---|---|---|
Time Frame | Often, product-based approaches assign the paper early in the semester with no touchpoints until the final product is due. A product-based approach to a research paper would assign the paper to students and then assess the paper students turn in, often at the end of the semester. | The “gold standard” of process/project-based learning necessitates sustained inquiry and opportunities for critique and revision. This means that there should be multiple checkpoints for the paper with corresponding feedback sessions. A process-based approach to a research paper would break down the task into a series of steps, providing feedback at each step. |
Authenticity | A product-based approach often assigns work that is a genre only used in a classroom setting. In this case, a traditional research paper. | Authenticity is often related to the audience. Who, beyond the teacher, is the audience of this work? How is this work appropriate for a task outside of the classroom? For a research project, this might look like submitting work to an academic journal or generating findings for outside stakeholders. |
Choice | The teacher assigns the form and content. There is limited choice– for example, students can choose from a few prompts. | Students generate the topic and final form of the work. |
Reflection | Cursory or no reflection | Students have multiple opportunities to reflect on their product and process. |
In summary:
- Explore AI for yourself.
- Establish and maintain an open dialogue with your students about GenAI
- Consider assessments that are informed by the affordances and limitations of GenAI.
Further Boston College Resources on AI
- CDIL’s Blog contains many posts on faculty experiences with AI in the BC classroom
- BC’s University-wide policies on Academic Integrity
- The Center for Teaching Excellence has an extensive section on teaching strategies for AI
- The BC Libraries guide for student use of AI